Advancements and Challenges in Genomic Medicine
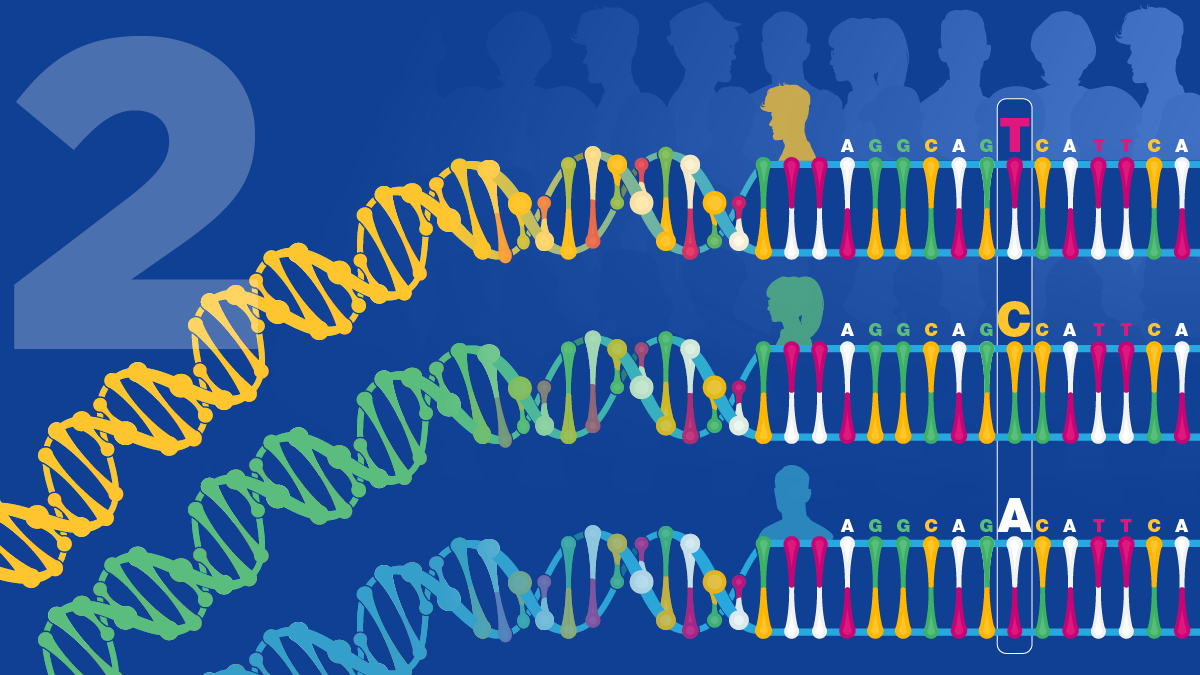
Fig.1 - Ref: https://www.genome.gov/dna-day/15-ways/human-genomic-variation
Some say that we should admit that genes are no longer the blueprint of life - https://www.nature.com/articles/d41586-024-00327-x; however, understanding their importance and impact on disease should not be
downplayed. We need to know how all the other factors and "life" elements interact and manifest healthy versus unhealthy systems - but we can also still learn a lot about the genetic impacts.
Were you aware that on the level of base pairs, your genome shares 99.9 per cent similarity with the genomes of other humans around you? (Fig.1). However, within that 0.1 per cent variation, many distinctive traits that make you unique can be found!.
Genetic variants are alterations in the DNA sequence that make individuals unique. The human genome, the complete set of genetic material, consists of about 3 billion base pairs of DNA organized into chromosomes. Genetic variants can occur at the level of a single nucleotide (single nucleotide polymorphisms or SNPs), or they can involve more significant segments of DNA. There are several types of genetic variants ranging from a single base pair change (SNPs) to structural variants involving larger DNA segments.
Genetic variants play a crucial role in the diversity of traits and disease susceptibility. Some variants are associated with specific characteristics or conditions, while others appear currently to have no apparent effect. Additionally, genetic variants can interact with environmental factors, influencing an individual's overall phenotype. Understanding genetic variants is essential in the fields of genetics, genomics, and personalized medicine. Advances in technology, such as high-throughput sequencing, have facilitated the identification and analysis of genetic variants on a large scale. This knowledge has implications for disease risk assessment, drug development, and personalized treatment strategies based on an individual's genetic makeup.
Variant classification is a critical component of clinical decision-making in a diagnostic setting. It involves the interpretation and evaluation of genetic variants to determine their potential pathogenicity. The American College of Medical Genetics and Genomics (ACMG) has recommended five variant classification categories: pathogenic, likely pathogenic, uncertain significance, likely benign, and benign.
Genomic medicine has revolutionized healthcare by providing insights into an individual's genetic makeup. Variant classification, a crucial aspect of genomic analysis, involves the interpretation of genetic variations to determine their significance in health and disease. The evolution in Bioinformatics techniques and genomic data analytics has facilitated the development of custom workflows which automate the variant analysis & classification process. Below is a sample high-level variant analysis & classification workflow.
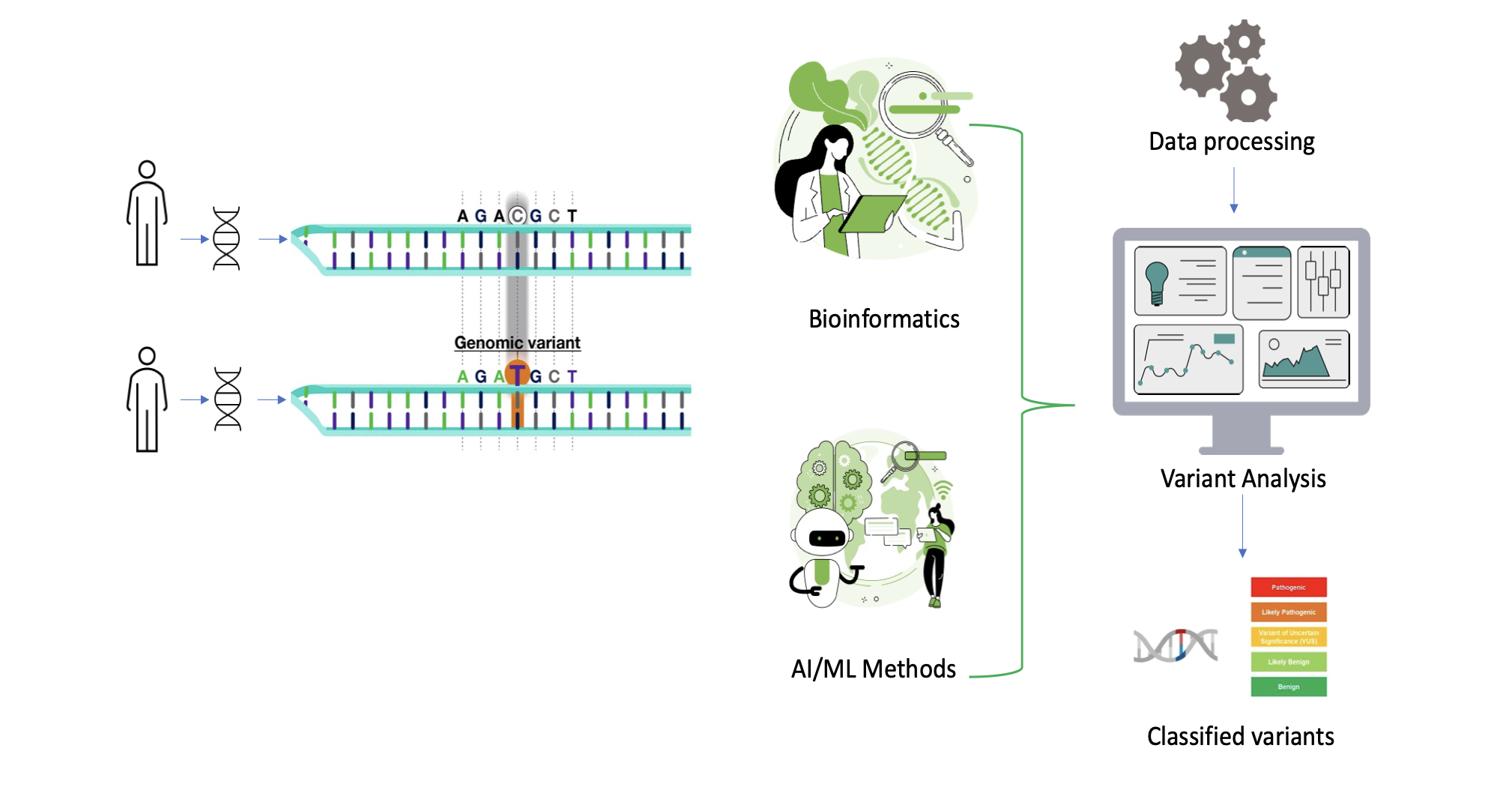
Fig2. Variant analysis and classification workflow.
Advances in variant classification have propelled precision medicine forward, but they also bring forth challenges that necessitate continuous research and innovation. Below is the overview for some of the topics in advancements and challenges in variant classification.
Advancements in Variant Classification:
1. High-Throughput Sequencing Technologies
- Recent years have witnessed remarkable advancements in high-throughput sequencing technologies, such as Next-Generation Sequencing (NGS). These technologies enable the rapid and cost-effective analysis of large genomic datasets, allowing clinicians to identify a wide array of genetic variants.
2. Public Databases and Resources
- The availability of extensive public databases, like the Genome Aggregation Database (gnomAD) and ClinVar, has significantly enhanced variant classification. These repositories provide a wealth of genetic data, facilitating the comparison of newly discovered variants with existing knowledge, aiding in their classification.
3. Machine Learning and Artificial Intelligence
- Machine learning algorithms and artificial intelligence (AI) have emerged as powerful tools in variant classification. These technologies can analyze complex genomic data patterns, identify subtle correlations, and predict the functional impact of genetic variants. The integration of AI into variant interpretation processes has expedited analysis and improved accuracy.
4. Functional Studies and In Silico Tools
- Advances in functional genomics and in silico prediction tools have contributed to a more comprehensive understanding of the biological effects of genetic variants. Functional studies help validate the impact of variants on gene function, while in silico tools predict the potential pathogenicity of variants based on computational models.
Variant classification and analysis involve a combination of tools and software that leverage bioinformatics, statistical methods, and computational algorithms. Here are some key tools and software commonly used in variant classification in a tabular format.
Table 1: Tools & Software applied in Variant analysis.
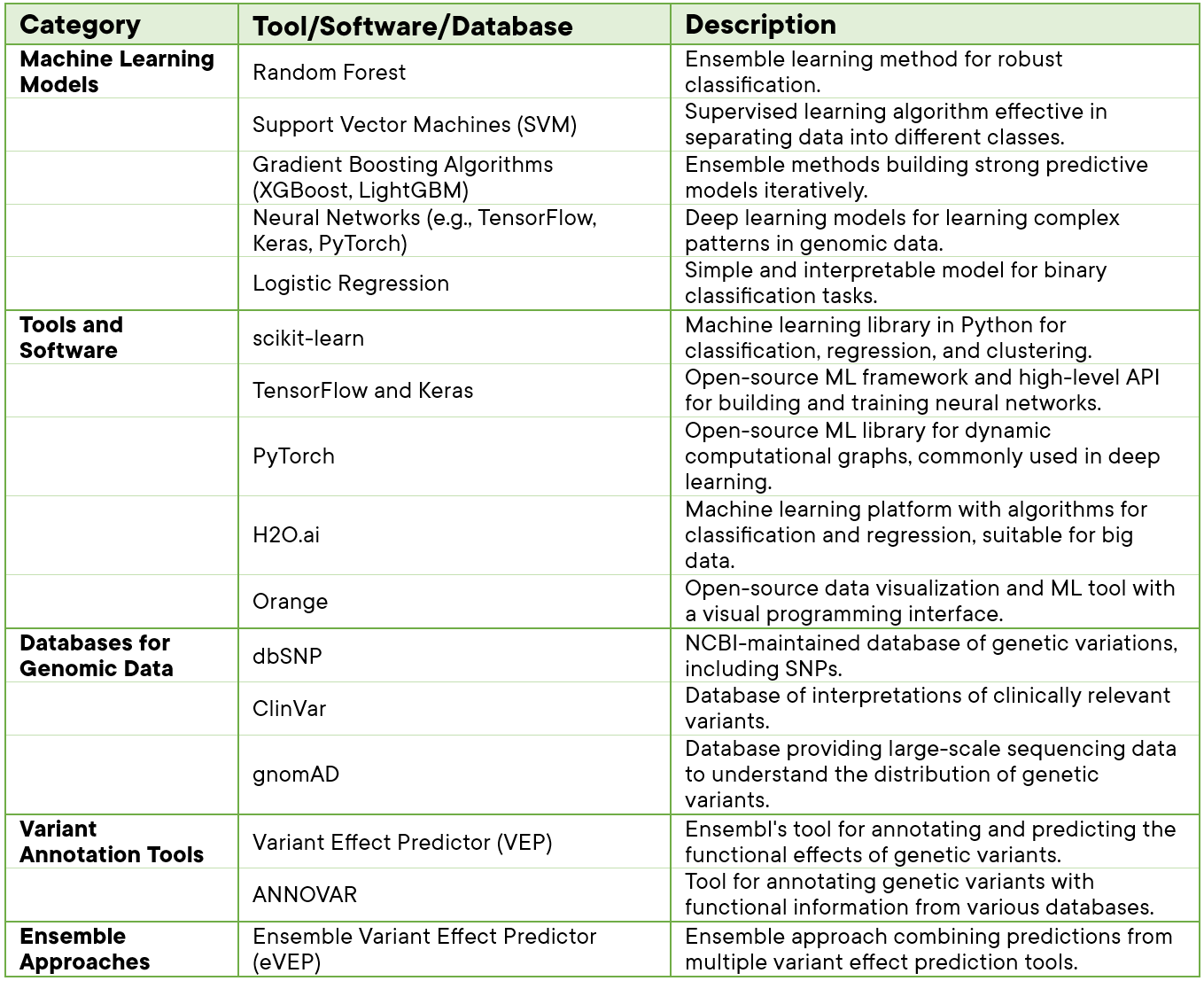
These models, tools, databases, and software (Table 1.) collectively contribute to the development of robust and accurate machine learning-based variant analysis and classification pipelines. The choice of specific tools depends on the nature of the data, the scale of the analysis, and the goals of the study.
Challenges in Variant Classification:
1. Variant of Unknown Significance (VUS)
- A significant challenge in variant classification is the presence of variants with unknown significance. VUS poses difficulties in providing clear clinical guidance, making it crucial to improve our understanding of these variants through further research and functional studies.
2. Ethnic Diversity and Population-Specific Variants
- Genomic studies have revealed the existence of population-specific genetic variants. However, the lack of diversity in existing databases can lead to challenges in accurately classifying variants, particularly in individuals from underrepresented populations.
3. Complexity of Polygenic Traits
- Many diseases are polygenic, involving multiple genetic factors. Classifying variants associated with complex traits requires sophisticated analytical methods and a deeper understanding of the interplay between various genetic factors.
4. Clinical Implementation and Interpretation
- Translating genomic data into actionable clinical insights remains a challenge. Clinicians must interpret genetic information accurately and communicate findings to patients effectively. This necessitates ongoing education and collaboration between geneticists, clinicians, and other healthcare professionals.
Apart from these challenges listed, there are others which are lack of standardization in variant classification systems and the sheer volume of variants that need to be classified.
Variant classification is a complex process that requires expertise and computational power. With the rapid advances in sequencing technology, clinical diagnostic laboratories are identifying an increasing number of novel variants. This makes it difficult to keep up with the latest research and to classify variants accurately and consistently. The interpretation of genetic variants requires expertise and computational power, which can be a challenge for some laboratories.
If you're interested in variant calling, analysis, classification, research, and development, contact us by email at info@zifornd.com, and we are eager to collaborate and discuss further.
Happy Discovery from Zifo Global Bioinformatics Team!